The Role of AI
Due to the ever-larger amounts of available data, the research field of Artificial Intelligence (AI) has produced several ground-breaking advances, especially in the field of Machine Learning. End-users often experience these advances only through smart applications (e.g., automatic object recognition, face recognition, text extraction and search in photo libraries; live transcription of teleconferences, automatic translation, autonomous driving) or smart assistants (e.g., Alexa, Cortana, Google Assistant, Siri).
However, the vast majority of modern Machine Learning applications are based on techniques that came into existence as early as the 1970s. Back then, they were known simply as “Artificial Neural Networks,” and today they have been extended to give way to the so-called “Deep Learning” revolution. Deep Learning approaches became especially prominent for both research and industry after outperforming all other competing methods on the ImageNet Large Scale Visual Recognition Challenge (ILSVRC) in 2012. That is why within the AviaTor project, we are exploring the use of state-of-the-art Deep Learning approaches to support LEAs processing the growing number of CSAM reports.
Challenges for machine learning approaches in criminology
Before going into the details of some of these past and future explorations within the AviaTor project, it helps to first look at the main challenges arising from the scope of the project, and the nature of reports. One of the common challenges for modern Machine Learning approaches is the dependence on large amounts of (labelled) data. Besides that, large models often require special hardware (GPUs) in order to train and run. In the context of AviaTor, the data contains illegal media files. Consequently, one of the requirements of the project is that the data cannot leave the infrastructure of the LEAs. This means that Machine Learning components that are going to be used have to be trained on-premises, and in highly controlled environments.
The resulting models should also be treated with a rigor similar to the data that was used for training. At the same time, the availability of training data is considerably limited. Investigators already struggle with a high workload that involves reviewing highly disturbing material, so any dataset labelling efforts should only happen after careful consideration. If possible, labelling should be based on past cases to reduce additional manual effort as much as possible. Another critical challenge is the need for interpretable and trustworthy approaches. Especially in the context of this project, investigators should be able to understand why a component comes to certain conclusions.
This is not only one of the preconditions for trustworthy AI, but also a necessity for identifying unintended biases and preventing them from arising, for example, from imbalances in the data distribution. Aside from trust requirements, these considerations also make it clear that any Machine Learning component, as well as the resulting overall AviaTor system, should always be understood and designed as a semi-automatic support system with continuous re-evaluation and adaptations of its components. This way, investigators remain in ultimate control of any decision.
In addition to these mostly technical considerations, legal challenges with an impact on data-driven approaches arise while trying to fight CSAM across several jurisdictions. One example of this is the availability of past report data. In some jurisdictions, data needs to be deleted after short timespans, especially if no case was opened. Another issue is that definitions for CSAM often differ between legal frameworks (e.g., age ranges). The methods developed for AviaTor need to be flexible enough to adapt to such differences.
Past developments in AviaTor 1
In light of these overall considerations, AviaTor phase 1 was the first step in the direction of supporting investigators in their daily work, by creating a report-reviewing interface with various scoring components. During this first project, we conducted a statistical analysis of the data types included in the reports available to the Dutch and Belgian police. As expected during the proposal phase of the project, a large part of the reports include images. This is why concerning supporting classifiers, we focused on state-of-the-art Deep Learning classifiers of the visual domain in this first project. When working with the participating LEAs in order to try and find potentially available labels for the existing image data in past cases, we found that the only readily available labels in the past were whether a reviewed image ended up in an opened legal case or not.
As a trade-off between the above-mentioned considerations-the readily available labels and the additional efforts for investigators to create better, more fine-granular labels-we decided to train a binary classifier in the first AviaTor project. For a given image, the goal of this first classifier was to predict whether a similar image in the past would have caused a legal case to be opened or not. The resulting image classification score (i.e., the classification probability of the true class) can then be used as one of many other scores in the resulting system. The positive evaluation results of this first classifier show that it can be a valuable scoring component for investigators. However, due to the lack of fine-granular labels, the transferability and interpretability of the classifier’s score are not a given. The classifier score is currently one of the items that the investigator uses in prioritising a report. The investigator can manually add the importance of this classifier score in the scoring module in AviaTor, ensuring that the classifier score impact is balanced with the other elements in the report to define the right priority. If instead, the classifier was to predict multiple attributes of interest, this could increase trust in the system by being more interpretable since individual attributes could be configured to be weighted differently, based on the evaluation of investigators.
Current and future developments in AviaTor 2
Based on the foundations of AviaTor Phase 1, one of the clear goals set for AviaTor phase 2 was to extend the work on the visual classification to more fine-granular labels. During interviews with several European LEAs, we identified a list of interesting properties of images that would be desired to be automatically detected in the image contents of incoming reports. Once detected, such properties could then be used to support investigators as parts of the flexibly configurable scoring system of the AviaTor system across different jurisdictions.
While the exact identified features cannot be revealed here for investigative tactical reasons, in the prioritisation of the current developments we apply current state-of-the-art techniques, and take into account the feasibility, as well as the necessity of additional labelling work for investigators. Along these lines, in the future, we would also like to investigate whether ideas of decentralised training of classifiers can be used to further reduce the individual labelling efforts of participating and collaborating LEAs. Apart from images, AviaTor phase 2 project further focuses on investigating the creation of machine learning components to help with the increasing amounts of reported videos and textual information. Textual information is especially interesting for investigators to identify certain activities around CSAM - like coercion or grooming.
Expanding from very basic keyword lists and their matching, research topics here range from the supported identification of keywords or more complicated, but common, patterns that could indicate coercion or grooming. Challenges in this area however arise from the variety of different textual data types in the reports (e.g., logs, chats, discussions, metadata, descriptions, documents) as well as the very different verbosity and multilinguality of some of the reporting platforms.
Several of the ideas and challenges were already addressed as part of the previously mentioned interview process. Similar to the analysis steps in AviaTor phase 1 for the visual domain, we are currently gathering the necessary statistics about textual data to be able to estimate feasibility, necessary additional labelling efforts as well as decide on the implementation order of more advanced text analysis and understanding components.
Conclusion and outlook
In summary, AviaTor explores how to make use of very recent developments in AI to support LEAs in their fight against CSA. Currently, these explorations focus on a better support to deal with the strong increase of visual as well as textual report content. Each of the newly developed “smart” components is only a small piece of the resulting AviaTor system to support investigators.
Despite always being accompanied by other components, the AI components are carefully evaluated as part of the development process already. This helps us to identify and counter potential biases as early as possible. Continuous re-evaluation and improvement of the AI components, as well as granular and interpretable results in the form of structured predictions, are some of the main goals. This will lead to an increase in the value of AviaTor as a human-centred decision support system assisting investigators in their decision-making process.
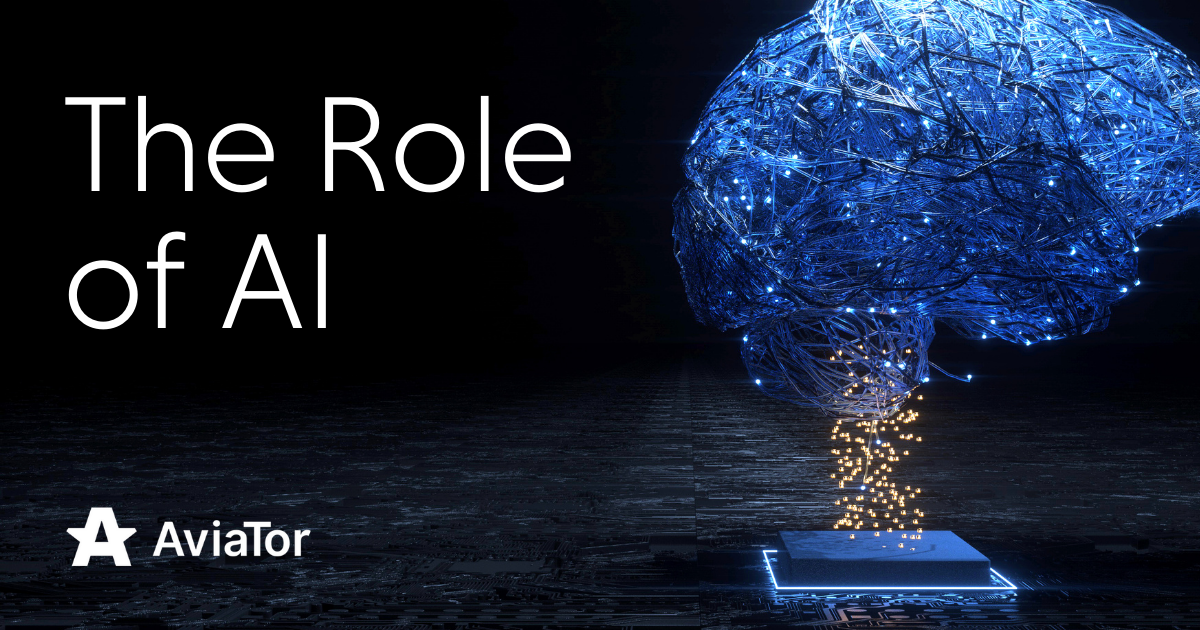
Any Machine Learning component, as well as the resulting overall AviaTor system, should always be understood and designed as a semi-automatic support system with continuous re-evaluation and adaptations of its components.
'